The essential role of natural language in representing biology
- samrodriques
- Dec 21, 2024
- 3 min read
At the time of writing, it is December 2024, and I am at NeurIPS. The word of the day, at least in the AI for Biology community, is foundation models. Everyone wants bigger data on more things to throw into bigger models. Virtual cell models will enable us to predict how cell states will change in response to chemical perturbations. Protein language models will enable us to identify better enzymes for degrading plastics or protein binders that have more drug-like properties. The future is bright.
Real biology discoveries look somewhat different, though, and I think it is telling that there are not very many actual biologists at these conferences. Contrast these dreams of foundation models with the latest table of contents from Science or Nature:
“A long noncoding eRNA forms R-loops to shape emotional experience–induced behavioral adaptation” — The authors identified a lncRNA in mice that is expressed in response to neuronal activity that modulates the 3D structure of chromatin, thereby activating genes that are involved in neuronal plasticity. The authors further identified that this lncRNA is essential for certain forms of learning.
“Cancer cells impair monocyte-mediated T cell stimulation to evade immunity” — The authors identified that mouse melanoma cells secrete a lipid metabolite that prevents monocytes from activating CD8+ T cells.
“Postsynaptic competition between calcineurin and PKA regulates mammalian sleep–wake cycles” — By generating mouse knockout lines, the authors identified phosphatases and kinases that are critical for regulating the sleep-wake cycle, and showed that they act through regulation of proteins at excitatory postsynaptic sites.
I struggle to imagine how any of these discoveries could fall out of a multimodal biology foundation model. This is not intended to be a straw man argument. Surely, a foundation model could potentially identify the lncRNA from the first paper, but I am not sure how such a foundation model would associate it with chromatin remodeling. A multimodal foundation model with enough data could also potentially identify metabolic changes associated with melanoma cells subjected to certain kinds of treatments, but I don’t see how that foundation model could identify the effect of those metabolites in preventing CD8+ T cell activation. Indeed, I do not think that any of the foundation models that are being developed today would be capable of generating rich new biological insights of the kind described in these papers. And yet, these are the kinds of insights that new therapies are made from.
The issue, I think, is that machine learning models work extremely well on structured data, and so all the foundation models that are being built are highly structured. Take a protein sequence as input and produce a protein sequence as output. Take a cell state and a chemical perturbation as input and produce a new cell state as output. Biology, however, is poorly structured. The lncRNA insight is case in point: what structured representation can we use for the action of the lncRNA in modulating chromatin architecture? Protein models cannot represent it; DNA models cannot represent it; virtual cell models cannot represent it. Perhaps a model that incorporates RNA expression and 3D genome state could represent it, but then how would that model represent the lipid modulation of the monocytes? I worry that every discovery may need its own representation space. Indeed, the nature of biology is such that there likely is no representation, short of an atomic-resolution real-space model of the entire organism, that is sufficient to represent the diversity of biological phenomena that are relevant for disease.
Except, of course, for natural language, which is evolved to represent all concepts that humans are capable of contemplating. Indeed, I think natural language is ultimately unavoidable for discovery in biology, insofar as it is the only medium we know of that is sufficiently structured for machine learning and sufficiently flexible to represent the full diversity of biological concepts. At FutureHouse, we work on language agents, which is one way of combining language and biology, but this is not the only way. Models that combine natural language with protein, DNA, transcriptomics, and so on will also be extremely productive, provided the addition of the structured datatypes does not restrict their ability to represent unstructured concepts.
The history of biology is built on tools that we have found in nature to study biological phenomena. As all biologists know, trying to engineer things from scratch (almost) never works; what works is finding things in nature and repurposing them. It will be aesthetically pleasing if it turns out that our engineered representations are yet again insufficient for studying biology, and that natural language is simply another such tool that we have found in nature that must be applied instead.
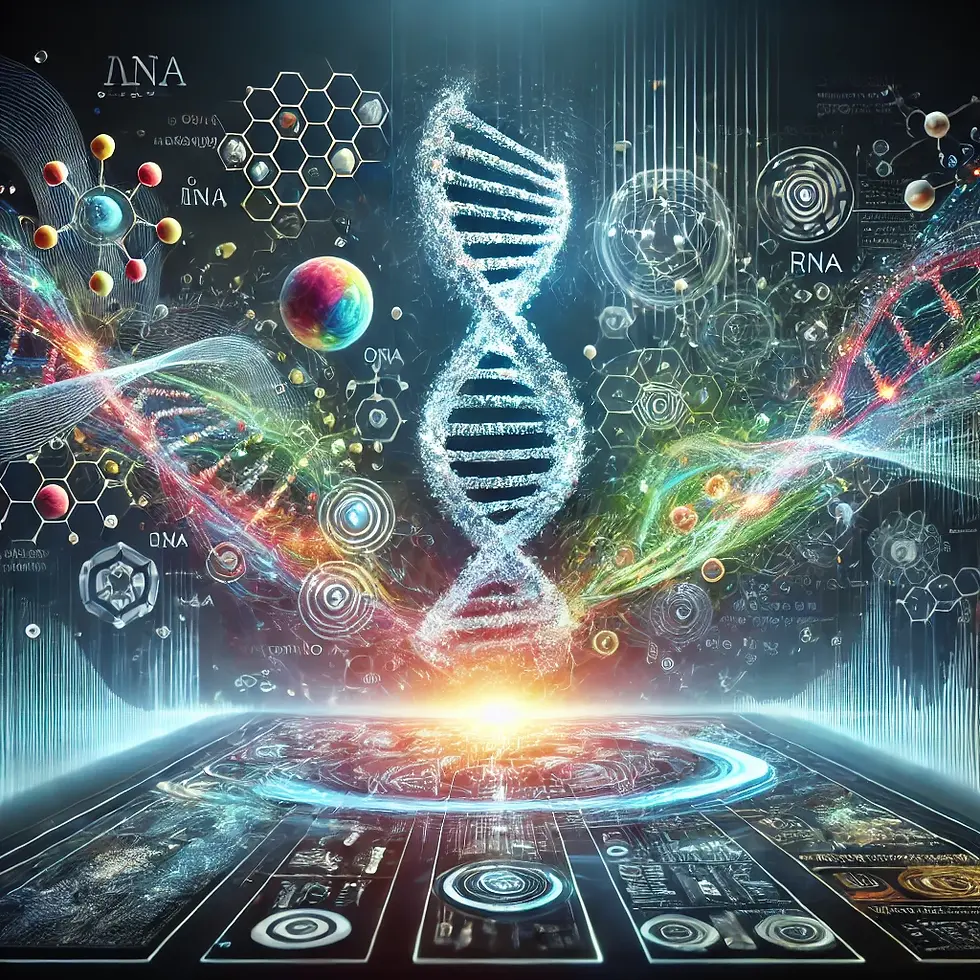
Professional movers are trained to handle items of all shapes and sizes. Whether it’s delicate glassware or heavy furniture, they https://ashevilleareamovers.com/ use the right tools and techniques to avoid damage. Their experience allows them to navigate tight spaces and protect items throughout the entire moving process.
A hallmark of Titan Research Group is its interdisciplinary team. Researchers from various domains, including social https://titanresearch.ca/ sciences, engineering, medicine, and economics, collaborate to tackle multifaceted problems. This fusion of diverse expertise promotes comprehensive and balanced research solutions.
Reliable healthcare staffing is critical for quality patient care, https://dsrecruit.co.uk and this agency delivers top-notch professionals. Their recruitment process is thorough, ensuring that medical institutions receive highly qualified candidates. Their efficiency and expertise make hiring stress-free.
Senior home care services in Manchester, CT, were exactly https://thehomeaides.com/ what our family needed. The caregivers were kind, knowledgeable, and reliable. They took great care in addressing my father’s personal and medical needs. It was so reassuring to know he was getting the best care possible while staying in his own home.
My cousin’s motorcycle crash left him with a busted leg and a https://galimidilaw.com/ totaled bike. His accident lawyer didn’t just fight for money—they made sure he felt heard during a rough time. The settlement covered his surgeries and gave him peace of mind. That kind of support is priceless.